Building safer AI for a better future
Marika Yang
Apr 25, 2019
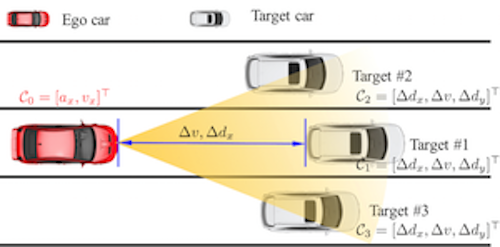
Source: Safe AI Lab
How do we define, classify, and model driving environments based on big traffic data?
Artificial intelligence has been a buzzing field in the tech industry for decades, but in recent years, AI has moved into the mainstream consciousness of technology and innovation. Amazon shopping recommendations, Gmail smart replies, and voice assistants like Siri and Alexa—these are all examples of AI in our everyday lives. But as AI continues to grow and research has expanded to products like autonomous vehicles, the question of safety is now at the forefront of this cutting-edge field.
Assistant Professor of Mechanical Engineering Ding Zhao wants to make AI better for everyone. He joined Carnegie Mellon University in Fall 2018 and currently leads the Safe AI Lab, spearheading research to develop safe, transparent, and reliable AI that is backed up by data and provable experiments.
“Safety is the ticket to enter the world; without safety, AI can go nowhere,” Zhao says. “$100 billion is being spent on AVs globally—a lot of resources are being put into it, along with academia support. We have many big problems, yet we do not have any official regulation for safety all around the world. There are high requirements in safety for machines, therefore automated vehicles are the perfect machines to use for my research.”
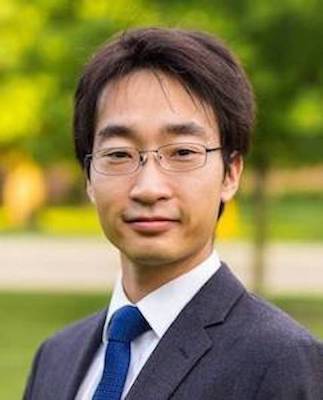
Source: Ding Zhao
Zhao joined the College of Engineering in 2018.
Building safe AVs is extremely complex. Even the top innovating companies, such as Tesla, Waymo, and Uber, have had difficulties with maintaining safety, with reported crashes each year. But why is it so hard to realize safe AVs on the road?
In his work with the Safe AI Lab, Zhao highlights two divergent obstacles that engineers and computer scientists much tackle. The first is that cases with safety issues are rare, whether they are minor collisions or fatalities. These are the cases we hear of on the news, but out of all the experiments, there simply isn’t enough data on these cases for AI technology to learn from.
“We use machine learning, for the AI to learn something,” he says. “However, the real thing it needs to learn is embedded in the corner of the gigantic data set.”
The second obstacle is how to describe the tasks for a certain operational environment. This process is very complex because the world itself is complex. Humans are unpredictable: we text or call on the phone (or do both), we get road rage, we daydream. On the road, there are jaywalkers and other pedestrians, there are cyclists, and other unpredictable drivers. Each driving situation is different and varies with time. We generate so much data, so much that it’s not efficient to analyze.
“The traditional methods that are model based methods cannot be efficiently used to analyze such a data set,” Zhao says. “We need to use some machine learning that automatically extracts information out.”
Safety is the ticket to enter the world; without safety, AI can go nowhere.
Ding Zhao, Assistant Professor of Mechanical Engineering, Carnegie Mellon University
Since arriving at Carnegie Mellon last fall, Zhao has been working to address these challenges. In the research project Accelerated Evaluation, he and his colleagues use methods from advanced statistics, modeling, optimization, control, and big data analysis to model this unpredictable environment using stochastic models.
He has also generated an unsupervised learning framework to analyze people’s driving behaviors, which can vary drastically according to many factors, including geography, by breaking the data down into small building blocks, or Traffic Primitives.
Zhao has partnered with companies and organizations to work on this research, including Uber, Toyota, Bosch, the Department of Transportation, and the City Council of Pittsburgh. In the future, the safe AI methods he is developing for AVs can translate to many other fields such as cybersecurity, regulation, and energy sustainability. He hopes his research helps pave the way for safer AI that in turn leads to a safer future.
Media contact:
Lisa Kulick
lkulick@andrew.cmu.edu